Imagine wanting to use the power of blockchain: secure, transparent, and decentralized but running into complicated setups, high costs and a steep learning curve. That’s the challenge many businesses face today. Blockchain as a Service (BaaS) offers a solution. It provides ready-to-use blockchain infrastructure through the cloud, so companies can build and run blockchain applications without managing servers or technical details. With BaaS, you can deploy smart contracts. Decentralized apps (dApps), and distributed ledges more easily and cost-effectively.
In this article, we’ll explain how BaaS works, explore its benefits, look at real-world use cases, and compare top providers in 2025. So you can see if BaaS is right for your business.
1. What is Blockchain as a Service (BaaS)?
Blockchain as a Service (BaaS) is a service that enables businesses to use blockchain technology without the need to build complex infrastructure. Instead of worrying about setting up and maintaining the system, you simply use the platform provided by cloud service providers like AWS or Microsoft Azure.
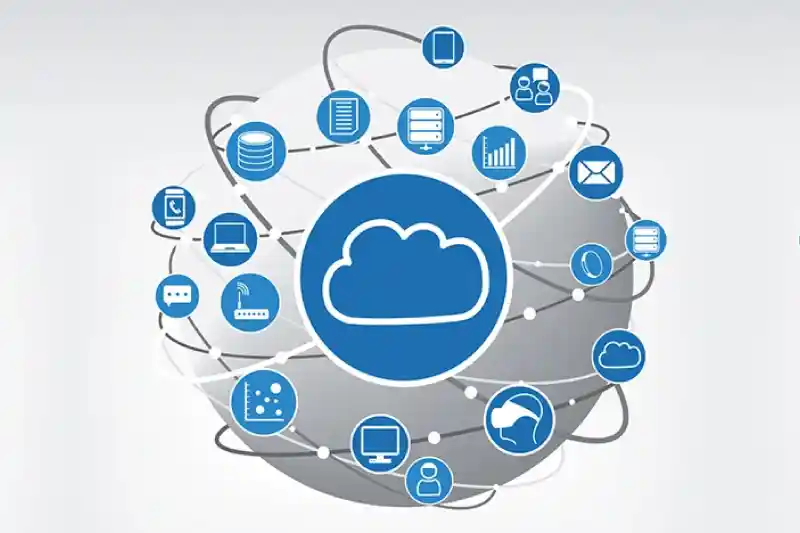
BaaS allows companies to easily deploy blockchain applications, such as smart contracts and decentralized applications (dApps), without needing an in-depth technical team. It’s like renting a ready-to-use system instead of building one from scratch.
This makes blockchain easier and more cost-effective, suitable for businesses of all sizes, and opens up opportunities for innovation in industries like finance, supply chains, and healthcare.
Read more >>> 15 Best Blockchain Programming Language for Smart Contracts and DApps
2. The BaaS business model
Blockchain as a Service (BaaS) offers businesses a way to access blockchain technology without the complexity of managing infrastructure. Leading BaaS providers like AWS, Microsoft Azure, and IBM offer ready-to-use blockchain solutions through their cloud platforms, making it easy for companies to integrate blockchain into their operations.
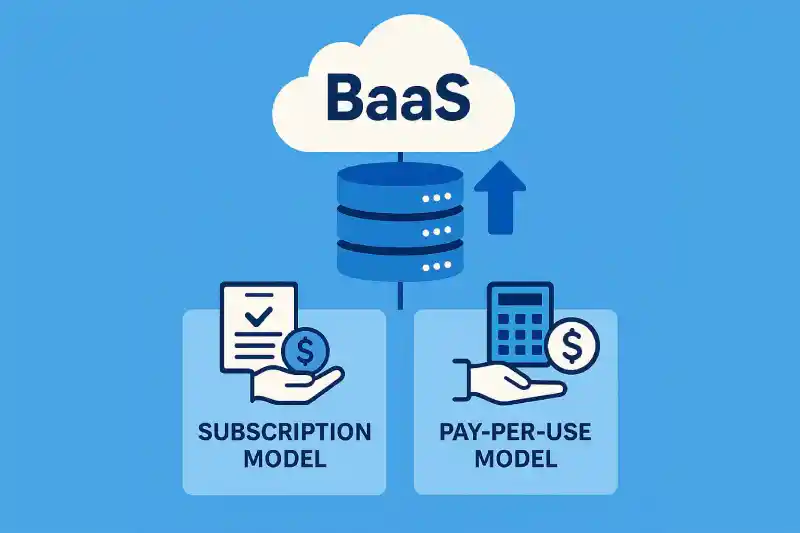
BaaS usually operates on one of these two business models:
- Subscription-based pricing: Businesses pay a fixed monthly or annual fee for using the BaaS platform. This gives them access to a set of services, resources, and tools they can use for their blockchain applications.
- Pay-per-use model: In this model, businesses pay only for what they use. This means they’re charged based on the resources they consume, like how many transactions are processed or how much data is stored on the blockchain. It’s more flexible for companies with varying needs.
BaaS platforms also offer scalability and customization, so businesses can adjust their blockchain services as they grow. Whether a company needs a simple solution or a more tailored system, BaaS can adapt to meet those needs.
Read more >>> How to Create a Blockchain: Build Your Own Secure Network Today!
3. How Blockchain as a Service (BaaS) works?
BaaS makes blockchain technology accessible to businesses by offering a ready-made solution through cloud platforms. Here’s a breakdown of how it works:
3.1 Cloud-based infrastructure
Instead of setting up and maintaining your own blockchain network, BaaS leverages cloud infrastructure. This means businesses can use the provider’s network, eliminating the need for physical hardware or specialized IT expertise.
3.2 Managed services
BaaS providers take care of the heavy lifting. This includes node hosting, smart contract deployment, and ensuring the blockchain is running smoothly. This allows businesses to focus on developing their applications, without worrying about the technical details.
3.3 Security and compliance
Blockchain is known for its security, but BaaS providers ensure that your applications meet industry-specific security standards and comply with regulations. They handle encryption, data integrity, and ensure the blockchain is secure and up-to-date.
3.4 Scalability
As businesses grow, so do their blockchain needs. BaaS platforms offer scalability, so companies can easily expand their usage as they need more resources, transactions, or storage without significant additional costs or setup time.
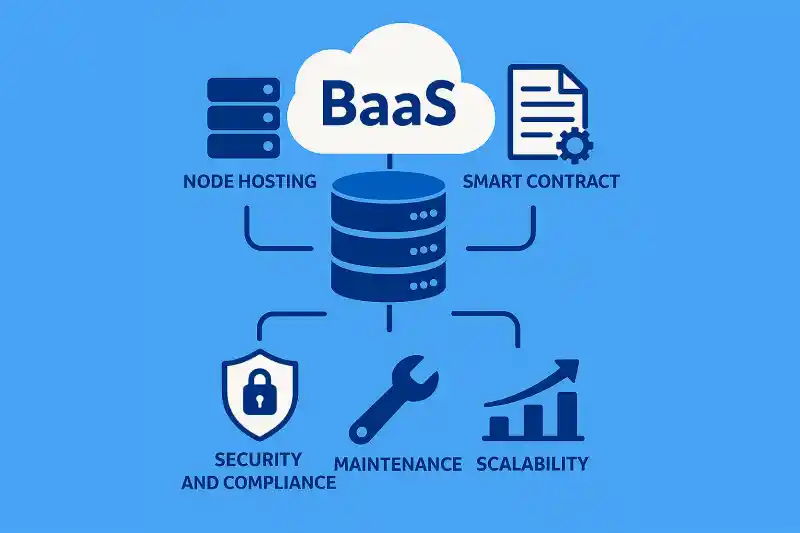
Read more >>> How Much Does It Cost to Create a Cryptocurrency in 2025?
4. Major players in the BaaS market
There are several key players in the Blockchain as a Service (BaaS) market that offer cloud-based blockchain solutions to businesses. These platforms provide the infrastructure, tools, and services needed to deploy blockchain applications quickly and securely. Here are the top BaaS providers in 2025:
4.1 Amazon Web Services (AWS)
AWS offers a range of blockchain services, including Amazon Managed Blockchain, which supports both Hyperledger Fabric and Ethereum. It’s known for its scalability, security, and flexibility, making it a popular choice for enterprises looking to build blockchain solutions.
4.2 Microsoft Azure
Azure Blockchain Service helps businesses quickly build and manage blockchain networks. Microsoft’s platform is known for its easy integration with other Azure services, making it ideal for companies that already use Microsoft products.
4.3 IBM Blockchain
IBM’s Blockchain Platform is built on Hyperledger Fabric and is widely used in supply chain, financial services, and healthcare. IBM provides strong enterprise support and customizable solutions to help businesses deploy blockchain technology.
4.4 Oracle Blockchain
Oracle’s Blockchain Platform is designed to help businesses create and manage smart contracts and blockchain networks. It focuses on integrating with existing enterprise systems, making it a good fit for large businesses that need to ensure compatibility with their current IT infrastructure.
4.5 SAP
SAP offers a range of blockchain services, with an emphasis on enterprise use cases such as supply chain management, traceability, and product verification. SAP’s platform integrates well with its other business software, providing a seamless solution for large companies.
Read more >>>> What Is Infrastructure as a Service (IaaS)? 3 Types of IaaS, Advantages, Disadvantages, How it Works?
5. Real-world examples of Blockchain as a Service applications
Blockchain as a Service (BaaS) is not just a theoretical concept; it’s already being used in various industries to streamline processes, improve transparency, and reduce costs. Here are some real-world examples of how BaaS is making an impact:
- Supply chain management: Blockchain technology helps track and verify the movement of goods in a supply chain, ensuring transparency and reducing fraud. For instance, companies like Walmart and De Beers use BaaS to trace products from their origin to their final destination, improving accountability and reducing inefficiencies.
- Financial services: In financial services, BaaS is used for cross-border payments and digital identity verification. Banks and financial institutions leverage blockchain’s transparency and security to enable faster and more cost-effective international transactions. HSBC and Barclays are examples of banks using BaaS to streamline operations and enhance customer experience.
- Healthcare data sharing: Blockchain can improve data sharing in healthcare by providing secure, immutable records of patient information. With BaaS, healthcare providers can share patient data across different organizations while ensuring privacy and regulatory compliance. Medicalchain, for example, uses blockchain to give patients control over their medical records.
- Voting systems: Blockchain can be used to secure voting systems, ensuring that votes are tamper-proof and transparent. Several countries have explored or implemented blockchain voting for elections and public referendums. West Virginia in the U.S. used blockchain technology for absentee voting in the 2018 mid-term elections.
Read more >>> What is Platform as a Service (PaaS)? Advantages, Disadvantages, Core Features
6. Benefits of Blockchain as a Service (BaaS)
Blockchain as a Service (BaaS) offers businesses a range of benefits, making it an attractive option for those looking to integrate blockchain technology without the usual challenges. Here are some of the key advantages:
- Reduced infrastructure costs: Traditional blockchain deployment often requires significant investment in hardware and maintenance. With BaaS, businesses don’t need to purchase or maintain costly infrastructure, as everything is handled by the provider. This makes it a more affordable option for companies looking to explore blockchain.
- Faster time-to-market: BaaS eliminates the need for businesses to build their own blockchain infrastructure from scratch. As a result, companies can quickly deploy blockchain solutions and start reaping the benefits sooner. Whether it’s for supply chain tracking, digital identity, or smart contracts, BaaS accelerates the time to market.
- Enhanced security and transparency: Blockchain technology itself is known for its security features, such as cryptographic encryption and immutability. BaaS takes it a step further by ensuring that businesses adhere to industry-specific security standards and compliance regulations. This makes BaaS a highly secure and transparent option for businesses.
- Scalability and flexibility: As a business grows, so do its blockchain needs. BaaS platforms are scalable, allowing companies to easily adjust their usage based on demand. Whether you need more storage, faster transactions, or additional smart contracts, BaaS can grow with your business, providing the flexibility you need.
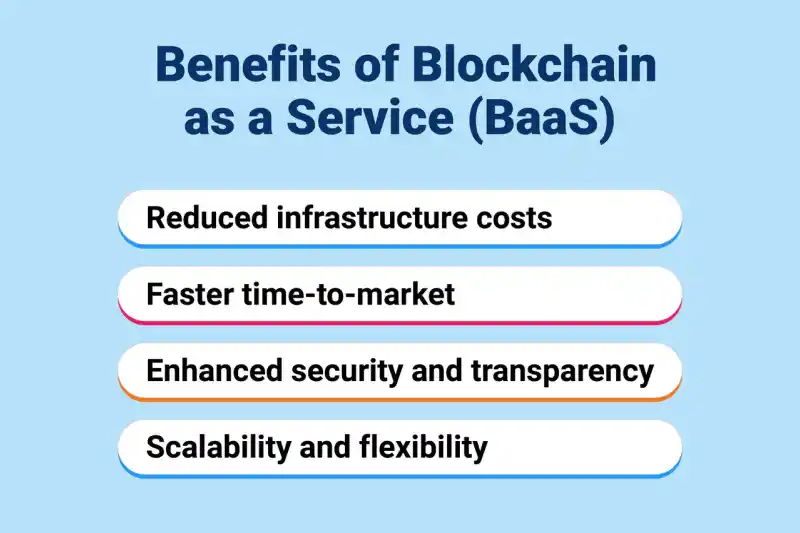
7. Challenges and considerations of adopting Blockchain as a Service (BaaS)
While Blockchain as a Service (BaaS) offers many advantages, there are also some challenges and considerations businesses need to keep in mind before adopting it. Here are some key factors to consider:
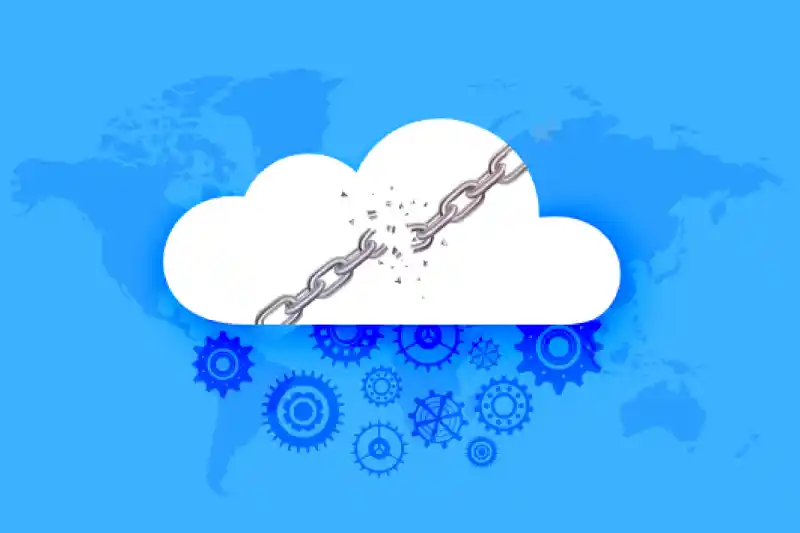
7.1 Vendor lock-in
When using BaaS, businesses may become dependent on a specific provider’s platform and infrastructure. This can lead to vendor lock-in, where it becomes difficult or costly to switch to a different provider in the future. It’s important to evaluate the long-term implications of choosing a particular BaaS provider.
7.2 Regulatory compliance
Blockchain technology is still evolving, and in many regions, the legal and regulatory frameworks around it are not yet fully established. Businesses must ensure that their BaaS solutions comply with local regulations, especially when it comes to data privacy and financial transactions. This is particularly important for industries like healthcare, finance, and government.
7.3 Integration with existing systems
While BaaS simplifies blockchain deployment, integrating it with existing enterprise systems can still pose challenges. Companies need to ensure that their BaaS solution works seamlessly with their current infrastructure and software tools. This may require additional customization or adjustments to existing workflows.
8. Conclusion
Blockchain as a Service (BaaS) makes it easy for businesses to leverage blockchain technology without the hassle of building infrastructure. It offers benefits like cost savings, faster deployment, and scalable solutions. However, businesses should consider factors like vendor lock-in and regulatory compliance before adopting BaaS.
If you’re looking to integrate blockchain into your business, Blockchain as a Service could be the perfect solution to unlock its potential. Ready to get started? Stepmedia can guide you through choosing the right BaaS provider for your needs and help you implement blockchain solutions that drive success.